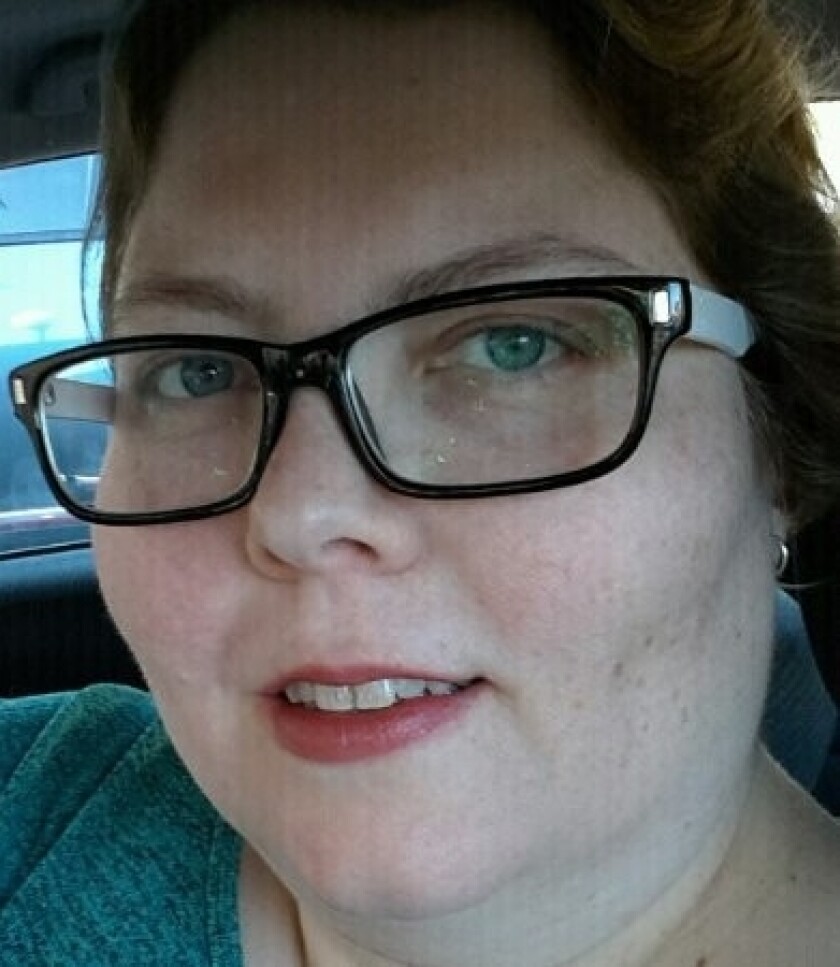
More than a decade later, we are still stuck on the second part of that saying. Data is dumped into massive repositories, and the expectation is that it will magically become useful. So, how do we transform all this raw data into something usable?
Back when disk space was expensive, we kept only structure data that was vital. There were data quality issues, but they were smaller in scope and size. Time spent classifying data was a money-saving strategy.
In the age of big data, it’s like we’ve become data hoarders. It’s not that more data is bad, but more data without cleaning is a mess.
Here’s another quote for you, from Forbes in 2016: “Data scientists spend 60 percent of their time on cleaning and organizing data.” In the world of data, data scientists are at the top of the food chain. These are the people who know what they are doing, and they are spending most of their time getting data ready for analysis.
This isn’t a task you give to someone who is untrained. Data analysis, and the subcategories of data quality and data categorization, are disciplines all on their own. The expectation that these skills are something any type of analyst has (like a business analyst or a compliance analyst or a policy analyst) is unreasonable.
For anyone who has tried to learn data analysis on their own, they quickly find it’s a dense subject. We’re talking about statistical methodologies in academic papers. Even after you’ve learned all the concepts, the next problem is translating them into practical steps. Someone can explain the idea of “duplicate data,” but defining what data is a duplicate in a dataset is specific to the business context of that dataset.
Throwing random analysts at large, unrefined datasets is a guaranteed route to data fatigue, analysis paralysis and bad intelligence. The alternative is to create a data culture at your agency. Start by accepting that data quality is an ongoing process, not a onetime project.
Consider hiring someone with expertise in the field of data to guide the process — like a data scientist, a research analyst or a data analyst. Consider external training for data analysis concepts and internal mentoring for data analysis practical application. Define your Key Performance Indicator (KPI) and give your data weight, so the ongoing cleaning and categorization of data is applied only to the important data.
A final saying: “How do you eat an elephant? One bite at a time.”
It’s time for you to consider what your first bite will be.