We already know that AI will augment and enhance everything — but there’s one major flaw in the design: What design?
Currently, we find ourselves in a fast-moving, technologically advanced world (note: as of this writing, some of the information in this article is likely already out of date). While artificial intelligence provides an evolutionary path into the future, it will cause some headaches along the way. A lack of standards and policies is a big part of the problem; there are some, but we have a long way to go. Being able to build training models and share them across solutions (cross-platform), and have policies in place, are essential in the evolution of AI use in government.
As nationally recognized AI guru Dr. Lisa Palmer recently pointed out to me, there is a lot of value added by vendors having proprietary large language models (LLMs). I would suggest we might also have a second model, or context engine, or even a hybrid model design that could provide agency-specific context — a well-defined, standardized solution that could be leveraged in a cross-platform environment. However, these well-defined models or context engines shared by solutions, platforms, and vendors do not exist today. This presents a big issue for large-scale solutions.
For smaller implementations, such as an HVAC Internet of Things (IoT) solution, for example, vendors’ proprietary models are very similar, and switching solutions is less of a concern. But when trying to implement large-scale systems — regardless of how — the unique AI training models somehow need to be shared with new solutions.
It is well-known that government agencies aim to serve the public by purchasing systems that both provide return on investment and serve a good purpose. Today the problem is that learning in a unique environment takes time (I call this the “spin-up” time of the solution). If governments are not able to better serve the public by acquiring new systems because of the AI spin-up delays found with proprietary models/learning, impacts will be observed in the cost-benefit. Every system has a slightly different LLM, but they all share a common structural requirement: a unique training model that is specific to the ecosystem in which the solution lives and can help provide agency-specific context and results.
If this learning can be captured by a standard secondary model or context engine design, it could be shared to a new solution. This would enhance flexibility when purchasing new solutions while maintaining vendor-proprietary models that pitch a product’s qualities. It is my belief this enhancement to the design would provide fewer contingencies and risks in deciding to change solutions.
It has become obvious that this is a problem, and OpenAI has recently announced a model specification to help. Although this is great, I believe a public entity should be responsible for developing this. I am not a fan of too much oversight, but I do believe it is necessary to help guide AI into an acceptable maturity.
In January 2023, the National Institute of Standards and Technology (NIST) released its first AI Risk Management Framework. This is another great start but still does not lead to much work in the realm of technical specification. ISO.org is also working on a project to help with AI guidance; you can read more about this here.
Another great initiative is the GovAI Coalition, spearheaded by the city of San Jose. The coalition aims to help public agencies at all levels of government below federal (see GSA for federal) build policies, templates, and base documents that other agencies can leverage as a starting point when building more regional policies.
In an ideal world, governments will be able to request proposals from vendors and reduce AI “spin-up” times by having these agency-unique models and context engines shareable across various platforms. We need to establish a base for AI technology before we dive too far off the deep end. Take, for example, the Von Neumann Architecture model for common computing: Yes, it has changed, but the basic concept has not. And fun fact: It was developed before the Internet!
Commentary: Industry, Government Should Speak Same Language in AI
“In an ideal world, governments will be able to request proposals from vendors and reduce AI spin-up times by having these agency-unique models and context engines shareable across various platforms,” writes Ben Palacio, senior IT analyst for Placer County.
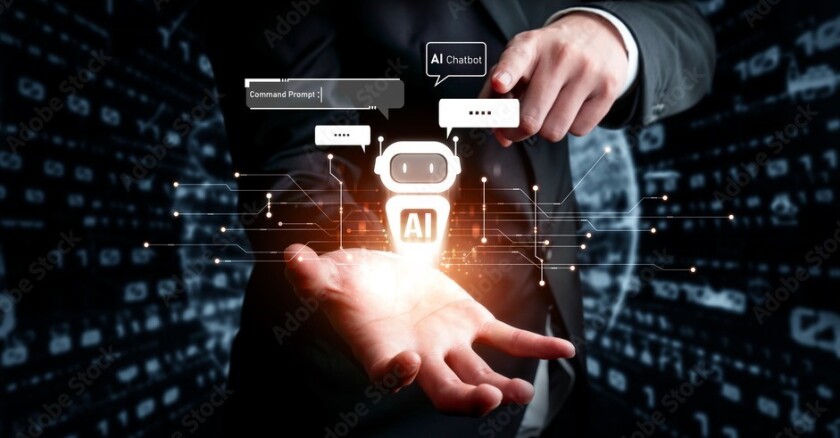